By Lars Bromley, Kersten Jauer, and Yossi Matias
Lars Bromley is a Specialist at the United Nations Institute for Training and Research – United Nations Satellite Center
Kersten Jauer is the Deputy Director for Strategic Planning in the Executive Office of the Secretary-General at the United Nations
Yossi Matias is the Vice President of Engineering and Research at Google
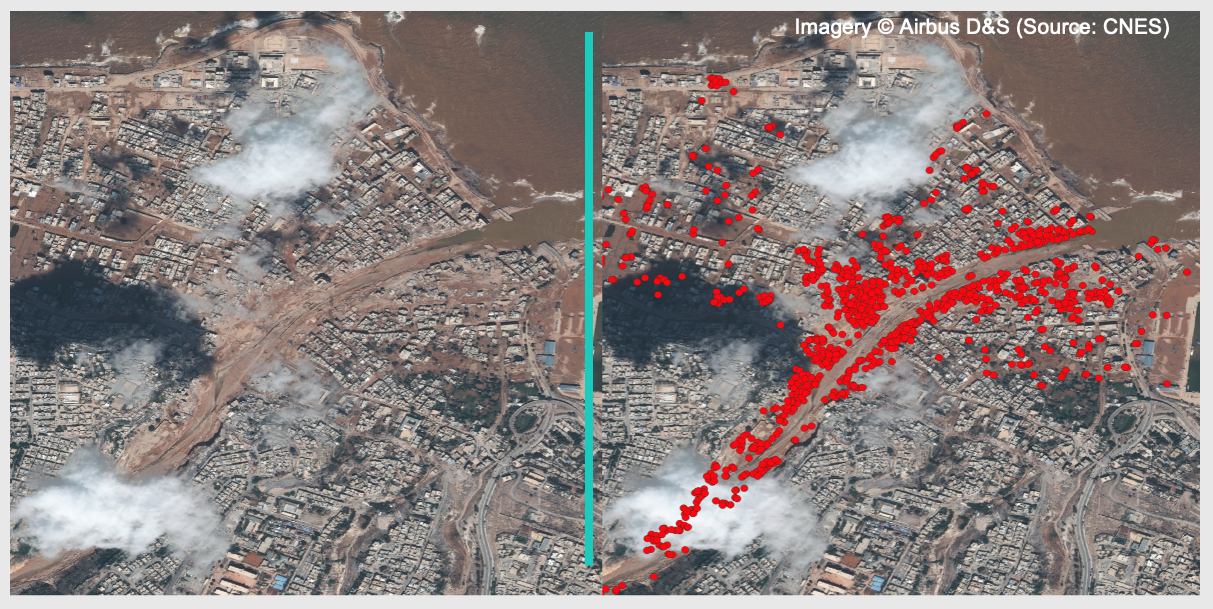
Between storms, earthquakes, floods, and wildfires, hundreds of natural disasters impact communities worldwide each year. Quickly identifying damaged infrastructure in the affected areas following a disaster is critical: the faster a high-quality analysis is available, the more disaster response efforts will be improved. When such analysis is needed, humanitarian agencies turn to experts from the UN Satellite Center (UNOSAT). These experts meticulously compare high-resolution satellite images from before and after the disaster to manually identify damaged buildings and infrastructure. UNOSAT’s analyses are widely used and respected throughout the humanitarian sector as the industry’s best. Most are made publicly available for the first responders around the world.
When disaster strikes, expert UNOSAT analysts work around the clock but often struggle to meet the growing demand for damage assessments. The time it takes to create these damage assessments varies based on the building density, geographic size of area impacted, the type of natural disaster and its severity. For particularly large and severe disasters, the manual assessment can be time-consuming. Humanitarian operations often cannot afford to wait for a comprehensive analysis of a full area, forcing difficult decisions about which areas should be prioritized for assessment.
To help address this growing gap, United Nations Global Pulse-led Data Insights for Social and Humanitarian Action (DISHA) coalition of partners joined hands with United Nations Satellite Center (UNOSAT) and Google Research to build an AI-enabled solution to assist human experts with damage assessments. The solution works on damages from earthquakes, storms, fires, as well as floods. The project team has tested the solutions’ performance on 9 recent natural emergencies, and found that, on average, the solution allowed the team to expand the area of analysis by a factor of 7, while reducing the time required to produce directional findings on damage assessment by a factor of 6, bringing it to under a day. This improvement in the speed of delivering directional assessments allows the team to perform assessments successively for crises that unfold over multiple days or weeks, allowing humanitarian organizations to better understand the dynamic evolution of the on-the-ground situation.
How does the AI-assisted Damage Assessment Solution Work?
The Damage Assessment solution makes use of three advanced models by Google Research: Open Buildings, SKAI Zero-Shot and the SKAI Fine-Tuned model.
- Open Buildings – Pre-trained vision model that can detect and segment building structures in high resolution satellite images.
- SKAI Zero-Shot – Pre-trained vision model that can identify damaged buildings in satellite images. The generated heatmaps can quickly highlight areas that may require priority in assessment and relief efforts.
- SKAI Fine-Tuned – Developed and open sourced in collaboration with the United Nations World Food Program (WFP) Innovation Accelerator, the supervised model can classify building damage in satellite images after being fine-tuned with images from the disaster being assessed and analyst-provided labels. This provides comprehensive information on damaged buildings.
Before damage assessment can begin, high-resolution pre- and post-disaster satellite images of the “area of interest” must be obtained. This process can take anywhere from less than a day to several days, depending on the size of the affected area and weather conditions – thick cloud cover can render the images unusable for analysis.
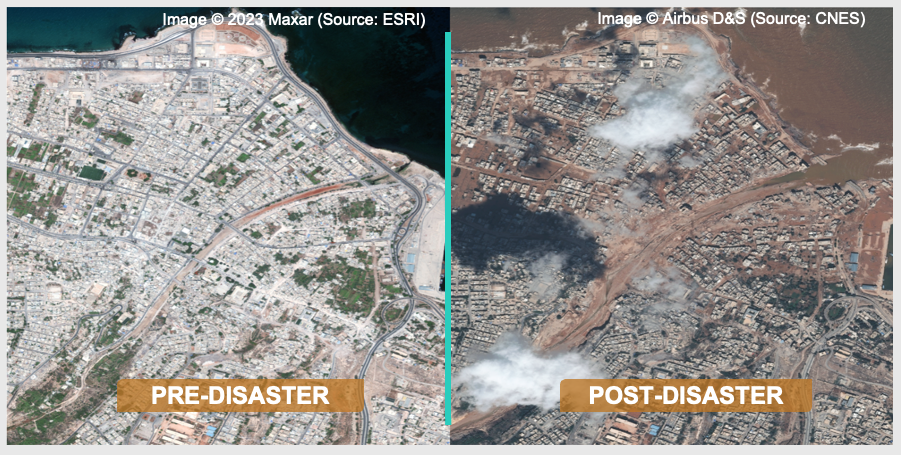
Figure 1. Pre- and post-disaster satellite imagery depicting severe flooding impact in an area.
Next, the image is overlaid with building footprint polygons generated from the Open Buildings model, or from sources such as OpenStreetMap.
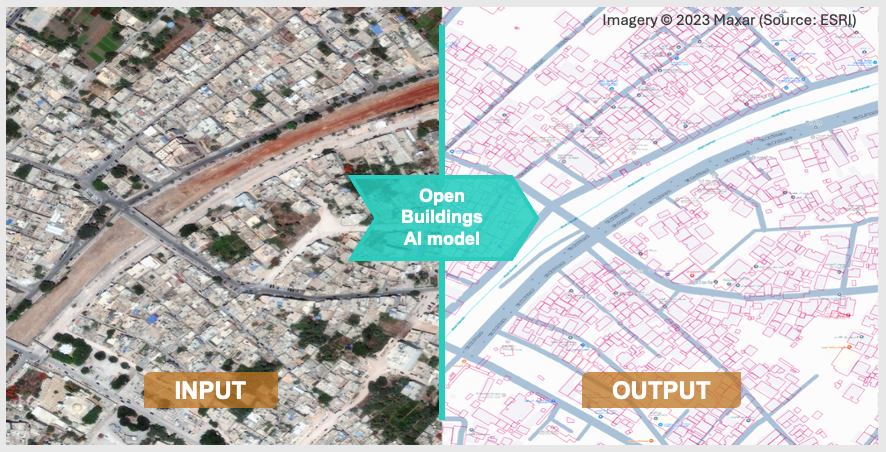
Figure 2. The Open Buildings AI model helps detect and segment building structures using satellite imagery.
Once the appropriate images and building footprints are obtained, expert analysts can use the DISHA Damage Assessment solution to run the SKAI Zero-Shot model providing a quick preliminary indication of the areas with the most building damage. This initial guidance can help UNOSAT analysts narrow their focus on specific areas for a more detailed analysis.
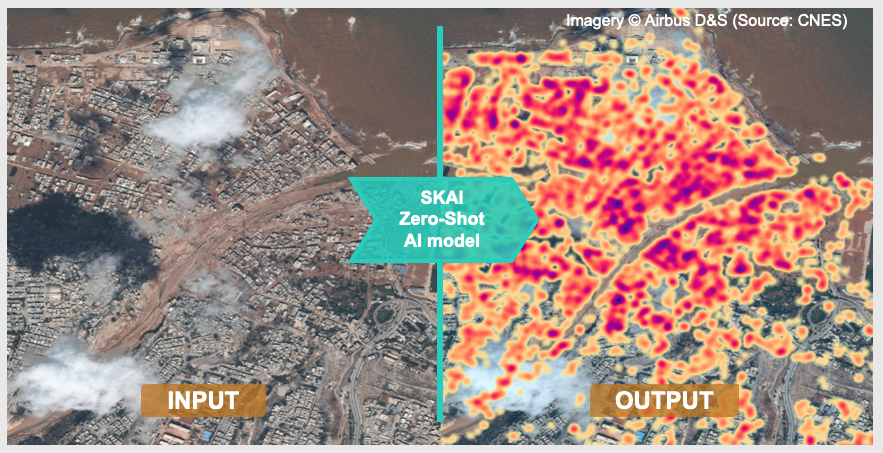
Figure 3. The SKAI Zero-Shot AI model quickly helps highlight areas of building damage using only post-disaster satellite imagery. Darker colors indicate higher levels of predicted damage
Once potentially damaged buildings have been identified, a stratified sampling approach is used to select examples for expert analysts, who then label the extent of damage by comparing pre-event images with post-event images.
Next, the labeled examples are used to fine-tune the SKAI model for the specific area of interest. The fine-tuned model is then deployed to assess the entire area, machine-classifying the damage levels of the remaining buildings – possibly hundreds or thousands – thereby saving expert analysts the time and effort required to assess each building manually.
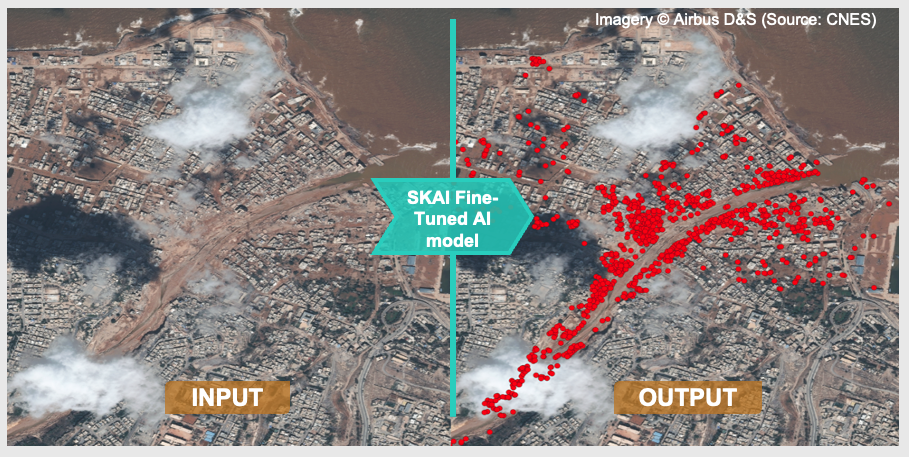
Figure 4. The SKAI Fine-Tuned AI model classifies damaged buildings (in red) in satellite images after being trained with disaster-specific images and labels provided by analysts.
Finally, the expert analyst reviews and, if necessary, corrects the model outputs before distributing the final damage assessment to humanitarian agencies via the UNOSAT website, ReliefWeb, and the Humanitarian Data Exchange.
Performance and Limitations
To evaluate the solutions’ performance, the team at UNOSAT compared the results of AI-assisted damage assessments with fully manual assessments across nine recent natural emergencies. February 2023 Marash/Antep earthquakes in Syria, September 2023 Adassil earthquake, May 2023 floods in Rwanda, September 2023 sea storm in Libya, July 2023 fire in Tunisia, December 2021 tropical cyclone RAI-21 in the Philippines, July 2024 tropical cyclone Beryl in the Caribbean, January 2022 volcanic eruption in Tonga, May 2024 floods in Bangladesh.
Our preliminary assessments of SKAI’s capabilities using UNOSAT damage assessment reports provided numerous insights. We found that, on average, the solution allowed us to expand the area of analysis by a factor of 7, while reducing the time required to produce directional findings on damage assessment by a factor of 6, bringing it to under a day.
When evaluating the accuracy of the SKAI output in identifying building damage at a granular level, we observed that the scarcity of damaged buildings relative to undamaged ones can hinder performance. In our tests, providing SKAI with a sufficiently large and balanced sample of damaged and undamaged buildings was key to achieving an accuracy of 0.75 and above (with 1 being perfect accuracy). Increasing the number of damaged buildings in the sample further improved the accuracy.
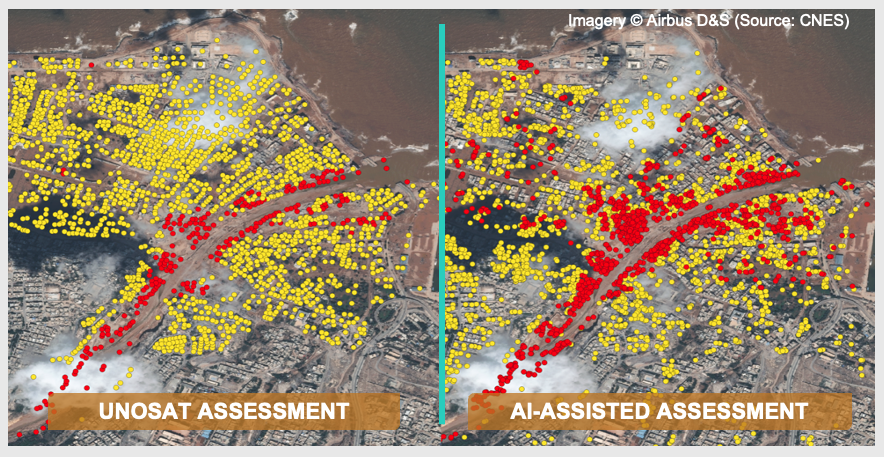
Figure 5. A sample assessment manually conducted by UNOSAT analysts, compared to an AI-assisted assessment that could potentially supplement and support their efforts in humanitarian disaster response. Yellow indicates potentially damaged buildings, while red indicates damaged buildings.
This post captures the results of testing the first version of the solution. DISHA will continue working closely with Google Research and UNOSAT to improve the solution’s performance and introduce new capabilities. We will also continue to evaluate and refine the process and solution over time.
Potential Future Areas of Collaboration
- Imagery Standardization: UNOSAT utilizes satellite imagery from various satellites, each with different formats and specifications. To maximize the effectiveness of the SKAI model, these images need to be standardized to an 8-bit depth RGB format. While this can be time-consuming, it is an important step in ensuring consistent and accurate results.
- Handling Insufficient Training Examples: As observed, our most effective models require at least 500 examples per class to perform well. However, some areas of interest in UNOSAT’s damage assessment reports may not provide enough examples to meet this threshold, limiting the model’s ability to learn effectively.
- Ensuring Representative Sampling: Understanding the underlying data distribution is crucial to ensuring that the sampled data is representative of the entire dataset. Addressing this challenge may help minimize biases and improve the model’s overall effectiveness.
The Coalition of Partners Driving this Work
Data Insights for Social and Humanitarian Action – DISHA is a multi-partner initiative led by UN Global Pulse, the Secretary-General’s Innovation Lab, and supported by a coalition of partners including Google.org, the Patrick J. McGovern Foundation, Jain Family Institute, McKinsey and Co., United Nations Development Programme (UNDP), and the World Food Programme (WFP). DISHA exists to help match advanced AI capabilities with humanitarian needs at scale by building and maintaining robust products that leverage relevant AI research, and facilitating collaborations among AI researchers, domain experts, and humanitarian organizations. The collaboration among DISHA, Google Research and UNOSAT on the AI-assisted Damage Assessment solution is our latest example of this model in action.
United Nations Satellite Center (UNOSAT) – We thank UNOSAT for providing critical domain expertise and playing a central role in shaping the product vision, debugging, and evaluating the solution’s performance. The partnership with UNOSAT is also core to ensuring responsible use of the solution: only expert analysts within UNOSAT who can spot and correct issues and inaccuracies if they arise have access; solution’s outputs will be quality-controlled by them before being distributed with the humanitarian users in strict compliance with UNOSAT’s security and data governance protocols.
Google Research – We thank the Google Research team for their hand-in-hand collaboration in developing, testing, and continuously refining the AI models and the Damage Assessment solution.
World Food Programme Innovation Accelerator – We are grateful to the WFP Innovation Accelerator for their valuable contributions in helping develop the original SKAI model in collaboration with Google Research.
We also thank InstaDeep‘s AI for Social Good (AI4SG) team for all their support to the SKAI open-source repository.
To learn more about the AI-assisted Damage Assessment solution, please email disha@unglobalpulse.org.
THE TEAM
UN Global Pulse
Strategy: Katya Klinova
Legal: Keepa Hipango, Vinesh Winodan, Caroline Alewaerts
Engineering: Tomaz Logar, Vanessa Maigné
Operation: Marco Scarpetta
UNITAR UNOSAT
Data Scientists: Alexander Kamper, Erika Gutierrez
Analysts: Jakrapong Tawala, Teodoro Hunger, Max Dean
Google.Org
Program Management: Aaron Ogle, Alex Diaz, Gabriel Doss
Google Research
– SKAI
Engineering: Andrey Mikhaylov, Jun Takahashi
Product: Waqas Burney, Moriah Royz, Tomer Shekel, Juliet Rothenberg
Operations: Beth Quisenberry, Deep Joshi, Matt Manolides
Partnerships: Chuthima Khaou, Gal Weiss
Legal: Amanda Ferber, Carl Fennessy
UX: Shruti Verma
– Open Buildings
Product: Tomer Shekel, Olivia Graham
Program Management: Abdoulaye Diack
WFP Innovation Accelerator
4UNOSAT’s assessments of non-sensitive areas are publicly available on the UNOSAT website and Humanitarian Data Exchange. Assessments of sensitive areas are shared with select humanitarian personnel only following the applicable security and data governance protocols.
5These examples were selected based on data availability and to evaluate the model’s ability to generalize across different landscapes and disaster types.